Automatic Classification of Non-Mass Breast Lesions in Dynamic Contrast-Enhanced MR Images
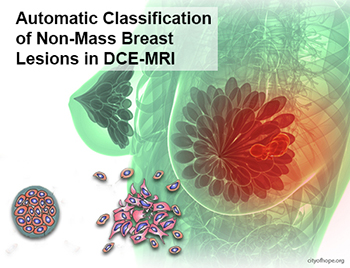
Today, breast cancer is the highest frequent diagnosed cancer and the most common cause of death among women. The early detection of cancer tumors via the screening programs is the essential policy to increase survival rates. Malignant cancer tumor, in its developed stage, can attack the surrounding cells and metastasis to distant body parts. Metastasis is the main cause of death in patients. In order to prevent that, the malignant cancer tumor has to be detected and treated in its early stage.
Description
Among lesion types the non-mass-like enhancements are the one with complex distribution patterns of enhancing tissue dispersed between normal tissues, thus their detection is not simple and more challenging than the masses. To fulfill this task, a semi-automatic mean-shift algorithm is used to segment totally 106 cases of non-mass lesions acquired from MR images of 86 patients (38 benign and 68 malignant). First, the segmented lesion volumetric object was packed using a sphere packing algorithm. Then, all sphere center coordinates were normalized. Next, a combination of different features was extracted from the internal spheres using various histograms, Zernike moments, and graph formation features. Finally, random forest machine learning is trained to be integrated into CAD (computer-aided diagnosis) system to do the classification.
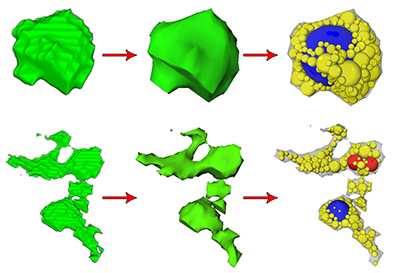
Sphere packing of a benign (top) and a malignant (bottom) lesion.
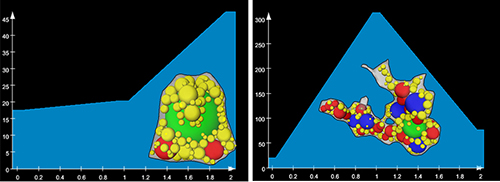
Volume-Radius histograms acquired from benign (left) and malignant (right) lesions.
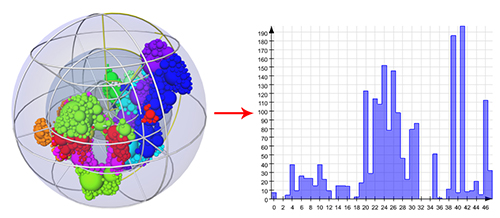
3D spherical wireframe and its corresponding shape histogram.
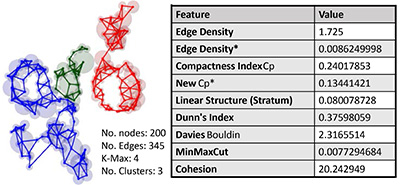
Graph topological features acquired from graph of connected center points.
Results
Classification is done using 10-fold cross validation technique in patient level over the ground truth dataset. In differentiating between malignant and benign lesions, an accuracy of 89.62%, precision of 90.1% and area under the ROC curve (AUC) of 0.972 is achieved using Random Forest algorithm by applying Mean Decrease Accuracy and Principal Component Analysis (PCA) feature selections.
The results suggest that the morphological features can be used for developing automated breast CAD for early detection of malignant non-mass lesions to achieve a high diagnostic performance. A higher accuracy can be even achieved, by taking advantage of morphological combined with kinetic, and texture features.
Files
Full version of the master thesis
Full version of the presentation slides
Here is a movie that shows features extracted from the packed lesions:
Collaborations
This work was performed in collaboration with Fraunhofer Mevis.
License
This original work is copyright by University of Bremen.
Any software of this work is covered by the European Union Public Licence v1.2.
To view a copy of this license, visit
eur-lex.europa.eu.
The Thesis provided above (as PDF file) is licensed under Attribution-NonCommercial-NoDerivatives 4.0 International.
Any other assets (3D models, movies, documents, etc.) are covered by the
Creative Commons Attribution-NonCommercial-ShareAlike 4.0 International License.
To view a copy of this license, visit
creativecommons.org.
If you use any of the assets or software to produce a publication,
then you must give credit and put a reference in your publication.
If you would like to use our software in proprietary software,
you can obtain an exception from the above license (aka. dual licensing).
Please contact zach at cs.uni-bremen dot de.